
The shipping industry has been working hard to tackle the shipping container shortage, which has seen products unable to be delivered, containers stranded at ports and containers being adapted for alternative use to assist with the problem.
However, although many companies have been working hard to combat the shortage, headlines across the globe are still highlighting this issue and what it could mean for the upcoming Christmas shopping rush.
In order to assist with this issue artificial intelligence company, MeetKai, has proposed a solution that will allow shopping trends to be identified ahead of time, allowing the industry to adjust its shipping container needs. The solution will also allow for situation testing, acting as a predictive means for the shipping industry to identify the best methods and plans of action.
We speak to MeetKai CEO James Kaplan to find out more about how this technology can assist the industry, and discuss the potential reasons why this technology has not been implemented sooner.
Could you provide me with some background on the company?
James Kaplan: My background is in finance, I ended up dropping out of college to start a hedge fund, trading options for a few years. In that space, trading options, especially quantitative finance are all about looking at interconnected patterns. You have all of these different strike prices, all this different expertise, it’s all about trying to find the correlated events between them.
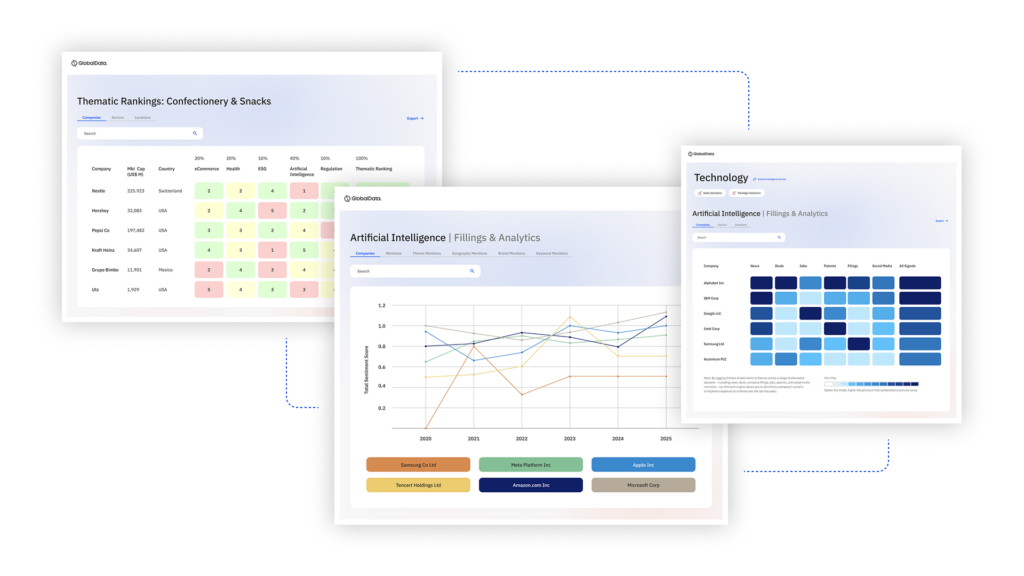
US Tariffs are shifting - will you react or anticipate?
Don’t let policy changes catch you off guard. Stay proactive with real-time data and expert analysis.
By GlobalDataIn options pricing, it’s entirely simulation-driven. You’re looking at how well these interconnected variables change over time, to find a better price. I really enjoyed that puzzle of looking at spaces like that, and that’s kind of how I got into starting MeetKai just to move into a more AI direction.
How could AI assist the industry with issues such as the shipping container shortage?
James Kaplan: The main way I think about the supply chain in general is that it is sort of a very beautiful web of interconnected parts, where a single bad element ruins everything. There’re few other areas where it involves interconnected parts, and one bad idea makes everything go down the drain.
When I looked at shipping, I thought this is an issue where you have all these different very correlated systems that are not talking to each other and being understood at a higher level. If you think about a really simplified version of supply chain, where you have manufacturers, shippers, retailers – way over simplified, but at the end of day, it does feel like the manufacturers are doing their own planning based on the orders they receive. The shipping companies are doing their own planning based on how many orders are coming through them. Retailers are also doing their own planning.
Every day I hear new explanations for why the supply chain is failing right now, it feels like no one has an answer, everyone just makes up a reason. In my mind it’s a lot of attempts to find the core elements, but it kind of makes me think that if this system were understood at a higher level, and you had higher level predictions, this wouldn’t have happened.
One of the examples I think about is they’re saying now that the price of cranberry sauce for Thanksgiving this year is going to be the highest in history. It’s very funny to think about that in hindsight, but to me, it seems like why couldn’t that be predicted? Why couldn’t it be anticipated? Thanksgiving is a holiday where people are using a lot of cranberry sauce.
There’s no communication, and I think that there’s a lot of companies trying to work towards that, but as I understand shipping industry, it’s very much specialised – you have hyper specialised companies that decide.
I think that AI is probably the only way to build a system that can understand both people and highly specialised companies.
Where would the AI be applied to assist these situations?
The main thing I think that would be interesting to me is if you could create an ensemble of models, where you predict the two things that really matter, which are: What is the cost to ship something going to be with these external variables? And what will the demand be of that item? These two form an equilibrium, because as the price changes because the shipping costs go up, that demand may go up or down.
In AI you can combine individual models into a meta model where it allows you to understand shipping costs and understand how demand works. Then you can learn the relationship between shipping cost and demand.
The nice thing about AI, and just in general about any type of machine learning, is that it’s very easy to test historically. We can go back in time and look at the price to ship a unit across the sea from any route that you want to talk about. We can build a model that predicts that and see how well it’s actually performing.
The most interesting type of models have different types of what’s called a holdout, where you say I’m going to show the model all the data from 2019, not show 2020, show it from three months in 2021 and see if it can fill in the blanks well. It is one of those things where I think focusing on just one of those two models is the first step. Once you have those two models, you would make the metamodel on top.
The nice thing about AI and these types of metamodels is that at the end of the day what you have is a system that can predict shipping costs, consumer demand, then the fun thing about AI is you can tweak the inputs. You can say if I have more cranes working, if I have the ports operating 24/7, will that reduce the shipping costs? It becomes a system where you can actually run simulations not just predict given the current state.
Why do you think that this technology has not been adopted sooner?
AI can be scary to develop, because there’s this sentiment that it’s going to put people out of jobs, when in fact that’s not the case. Almost every industry that’s had AI applied, hasn’t gotten smaller, it’s gotten larger. The second one is I think that in the entire supply chain, I’d say a hesitancy to share your pearls and let other people know what’s going on with your own model, everything on price model, no one really wants to give that information away.
The third reason is that AI is hard because until you get it to be good it can look really bad. There’s a very fine line between a model that’s actually learning and a model that’s just overfitting, and overfitting is the most dangerous thing for an AI because all it learns is how to replicate the past. If you had a model that perfectly knew the supply chain and then Covid-19 happens, it could just go off the rails into a completely different direction, because it doesn’t actually understand anything, it just knows the steady state.
I think we see that a lot with model AI everywhere. There’s the entire joke of one of the self-driving car companies has issues with puddles where it can’t drive well because they only tested it in the desert. That’s why AI gets risky.
I think that most likely the outcome of this is going to be that it’s going to be a necessity, and it can’t be something that’s avoided because all we really learned from the shipping container shortage (I think from an outsider to the industry) is that no one was prepared. It feels like on a day-to-day basis, you hear politicians making blind guesses as to what to do.
In the workplace AI has the biggest impact when a 5% improvement is a giant improvement. In a lot of industries, that’s not a big thing, that’s not noticeable. In some though, 5% is massive, if our supply chain was 5% more efficient, it could have huge cascading effects down line. So, it’s unfortunate that there’s that resistance.
Do you see this technology being the future of the industry?
I think it’s possible. What I see right now is that next year, my guess it’s that the industry will still be dealing with our current state and the fallout of it, then the industry will have to have that reflection moment, where it says: “How could we have prevented this, or how can we have recovered from it faster?”
I think depending upon which one they focus on will impact if AI becomes a staple or not. I think if they think about how we could prevent it then AI is in my mind the obvious answer.
However, if they think how they could recover from it, then AI might be delayed because they might look towards more policy changes. Hopefully, that’s not what happens, because I think we all like to think of industry and enterprises instead of recovering from bad situations, they should prevent them – but I think often at times it depends on who’s at the top making that decision.